In just 18 months since ChatGPT's debut, the largest technology companies in the world—including Open AI and other generative AI pioneers—have flooded the landscape with innovative AI-powered products. As soon as the market begins exploring the capabilities of a new chatbot or application, an even more advanced or cost-effective solution emerges that demands our attention. If you feel like this rapid wave of technological advancements is drowning you, you are not alone.
At ICONIQ Growth, our Analytics team aspires to collect and curate the latest information to help our community understand emerging trends, establish benchmarks, and plan for the future. Yet we found surprisingly little data exists about the early adoption, impressions, and preferences of enterprise buyers for this recent explosion in generative AI products.
To fill this gap, our team spoke directly1 to leaders within our community, including CDOs, CIOs, and CTOs to learn more. Combined with the insights from Bain’s GenAI Q1 2024 survey2, we dive into the evolution of generative AI and look forward to sharing more insights on this topic moving forward.
In our conversations with executives, we did not find a clear consensus on how companies are getting started with generative AI, what a future-proof tech stack should look like, or how to effectively measure a return on investment.
What we found was rather sobering: the vast majority of enterprise buyers are still developing their vision of the future and when or how generative AI might change their businesses. Most buyers do not yet feel confident they can predict what is coming or what will happen, even in the near term. In short, everyone is still searching, studying, and experimenting. However, we did walk away from the study understanding more about the ways in which these enterprise buyers are using generative AI, what sort of budgets they’re allotting for these efforts, and what solutions they are looking. A few notable takeaways:
- Adoption in the enterprise is still relatively nascent despite high demand.
- There are many barriers to entry, including lack of technical expertise, privacy concerns, performance, scalability, and cost for data and infrastructure.
- Buyers want to see real, measurable impact, and they are not seeing enough of it yet.
- Buyers are hungry for more. They are looking for a plethora of solutions that work in industry verticals and specific roles including, for example, finance.
- More companies expect to buy rather than build their own solutions.
- Funds are mostly coming from existing budgets originally earmarked for other purposes, making it a competitive environment for both incumbents and new generative AI entrants competing for limited dollars.
We are also left with some known unknowns, such as: Will the excitement of the past 18 months translate into repeatable sales, or was it just a curiosity mirage? Are people just playing with these new technologies for novelty’s sake or have they found enough value to go deeper and buy more?
The good news is this is not a “winner take all” market. There are many pockets of opportunity for solving specific use cases. ICONIQ Growth are enthusiastic participants in this new era. We plan to cover the State of Generative AI adoption on a regular cadence, so tune in here for the latest.
The State of Generative AI Adoption [Q1 2024]
Growing revenue, increasing operational efficiency, and driving employee productivity are the leading goals that enterprises are hoping to accomplish with generative AI.
“It doesn’t matter what you use. Nobody really cares what LLM you pick. What we care about is how it drives business outcomes for the business units that are allocating budget towards AI”
- CDO of Financial Institution1
That said, there are a number of concerns impacting adoption including lack of in-house expertise, quality and accuracy, data security/privacy, and the unproven ROI of generative AI. Another key consideration is that much of the underlying infrastructure (i.e., data silos across on-premise and cloud, lack of clean and labeled data, complex data infrastructure) at mature non-tech organizations is not in the ideal state to embrace generative AI.
“So much of AI innovation and adoption in the enterprise will be tied to organizational structure and how much managers empower their teams to experiment and figure out what works”
- CIO of Professional Services Firm1
Adoption of generative AI in enterprises has continued to increase since 2023, with 87% of respondents adopting generative AI solutions in some capacity3. The most popular use cases to date include natural language interfaces, software code development, customer service, and solutions to drive performance enhancements in products or develop new products/services.
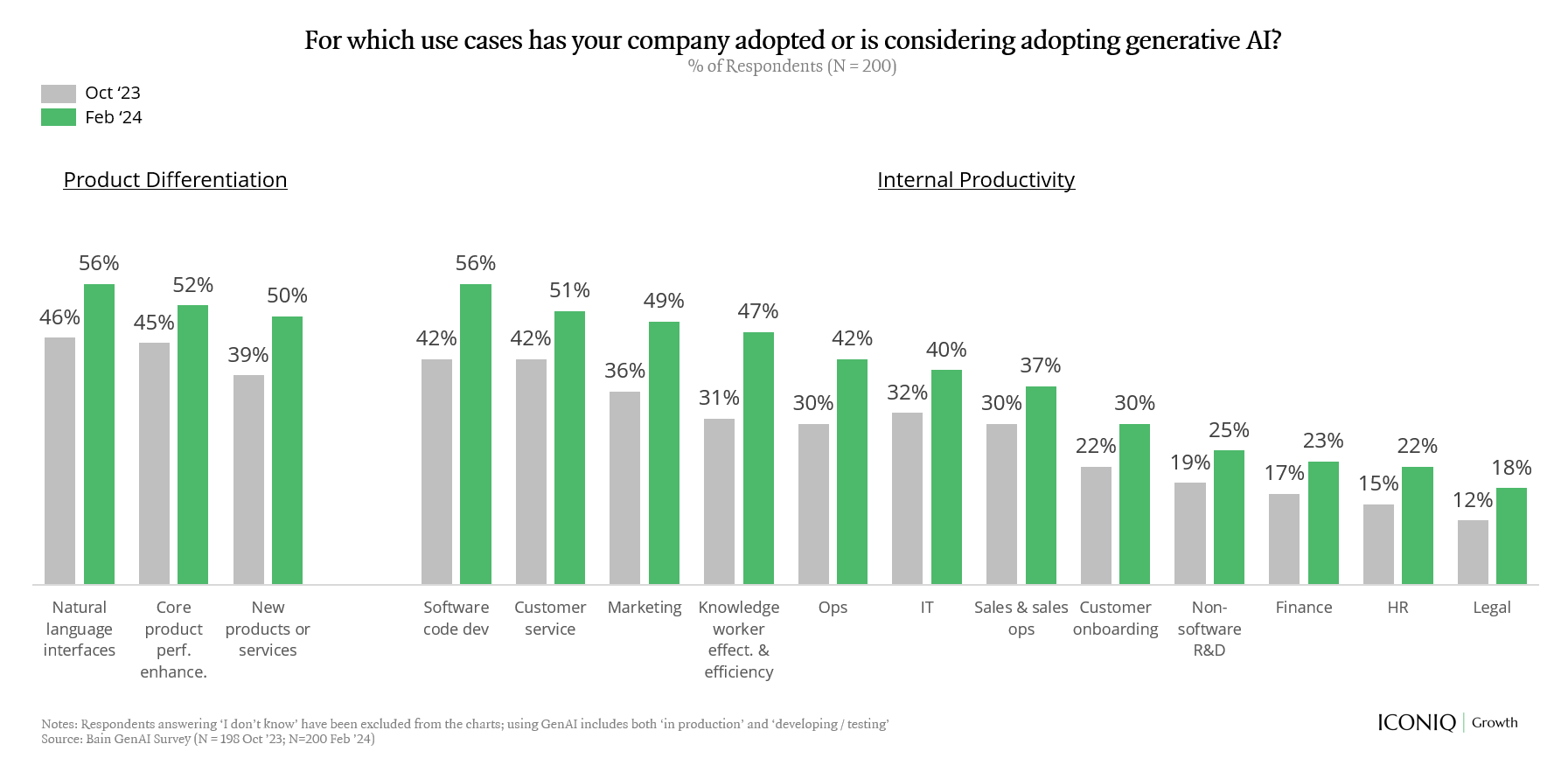
Technology firms are farther along in the deployment lifecycle across select use cases compared to firms in non-technology industries like financial services, healthcare, consumer goods, manufacturing, etc.3 This is likely driven by compliance and data security constraints in more regulated industries, in addition to a lack of readiness to adopt AI given the legacy technology and data architecture still prevalent in industries like healthcare and financial services.
“The F500 is not where we will see rapid adoption of innovative new generative AI tools (beyond widely adopted tools like Copilot). These orgs move too slow to adopt and implement in a space that’s changing so fast”
- CDO of Healthcare Provider1
However, across both tech and non-tech buyers, a limited percentage of use cases are actually in production.

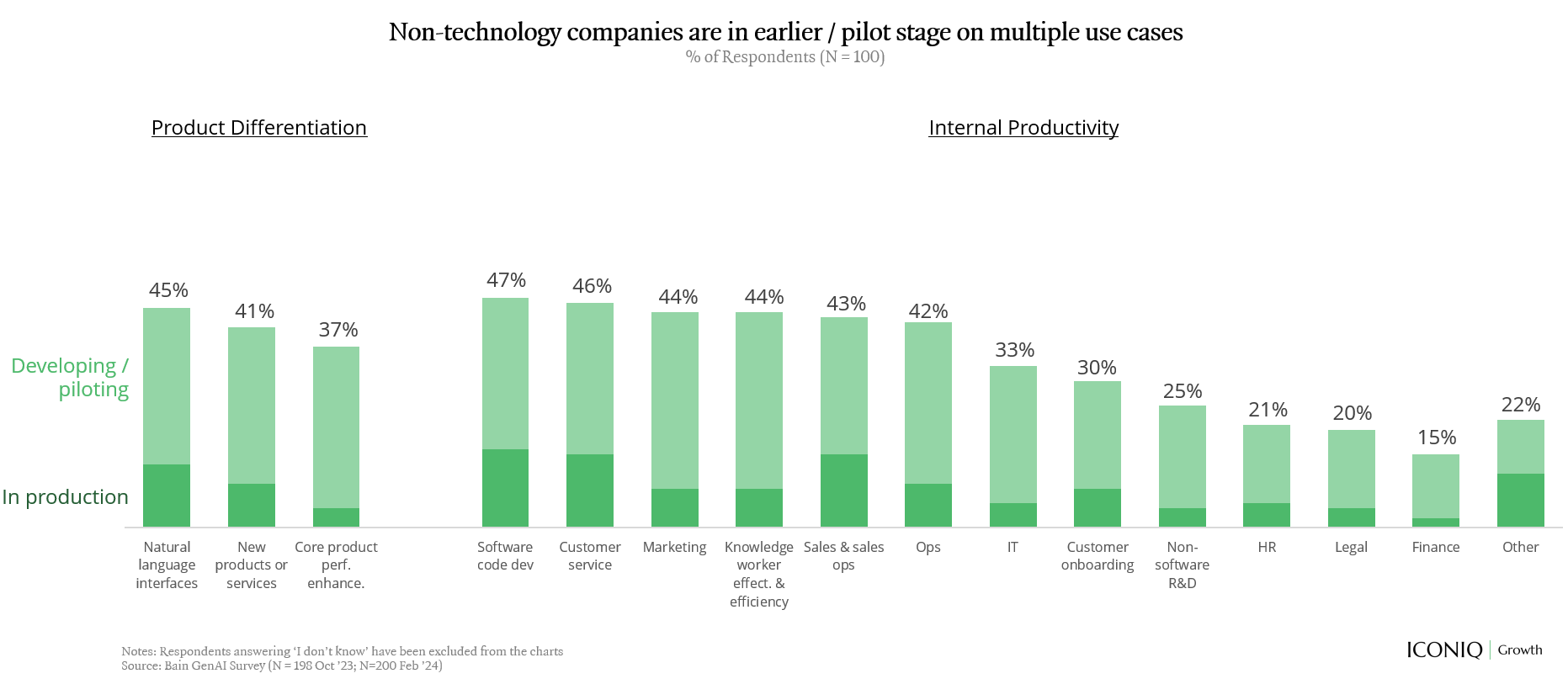
“Most of the investments in generative AI tools across the enterprise will be sunk costs because of issues with the quality of data. Generating LLMs on top of data sets with outdated and bad data will lead to hallucinations and bad outcomes”
- CDO of F500 Company1
AI Budgets
According to Glean’s5 latest CIO research, budgets for generative AI tools by IT teams will likely triple by 2025. Interestingly, cost efficiency, often a primary concern for CIOs when defining budgets, now ranks lower on the list of apprehensions regarding generative AI implementation. Only 18% of respondents surveyed by Glean consider the cost of third-party services a top concern, followed by 14% who are worried about the cost of developing or training large language models (LLMs).
In our conversations with CXOs, most of the dollars for AI budgets are coming from existing budgets being reallocated rather than net-new dollars.

Model Selection
As of February 2024, OpenAI is the most used foundation model, having been adopted by ~65% of respondents. Models from Amazon and Google followed, which have increased in adoption since October 2023 along with Meta’s LLaMA 2.

Unsurprisingly, the choice of models used also varies slightly based on the cloud service provider utilized by respondents, with companies on GCP or AWS more likely to utilize their respective foundation models (i.e. PaLM2 for GCP, Titan/Bedrock for AWS) in some capacity.
A key question companies are grappling with as they decide to embark on their respective generative AI journeys is the decision between build vs. buy. The majority of companies (~64% of respondents) seem to prefer some variation of the build approach—whether via model-as-a-service, finetuning, or proprietary models—compared to buying something off-the-shelf. Notably, this percentage has come down slightly from 68% in October 20234 which may signal slightly more openness to buying purpose-built solutions from external vendors, a dynamic we will dive into in future posts. And when considering technology vs. non-technology industries, there predictably tends to be a bias towards buying amongst non-technology respondents.
“You need to challenge people who want to build everything. If it’s not a core competency and it’s not going to yield a competitive advantage for the company, let’s buy and just build on top of a solution”
- CIO of Tech Company
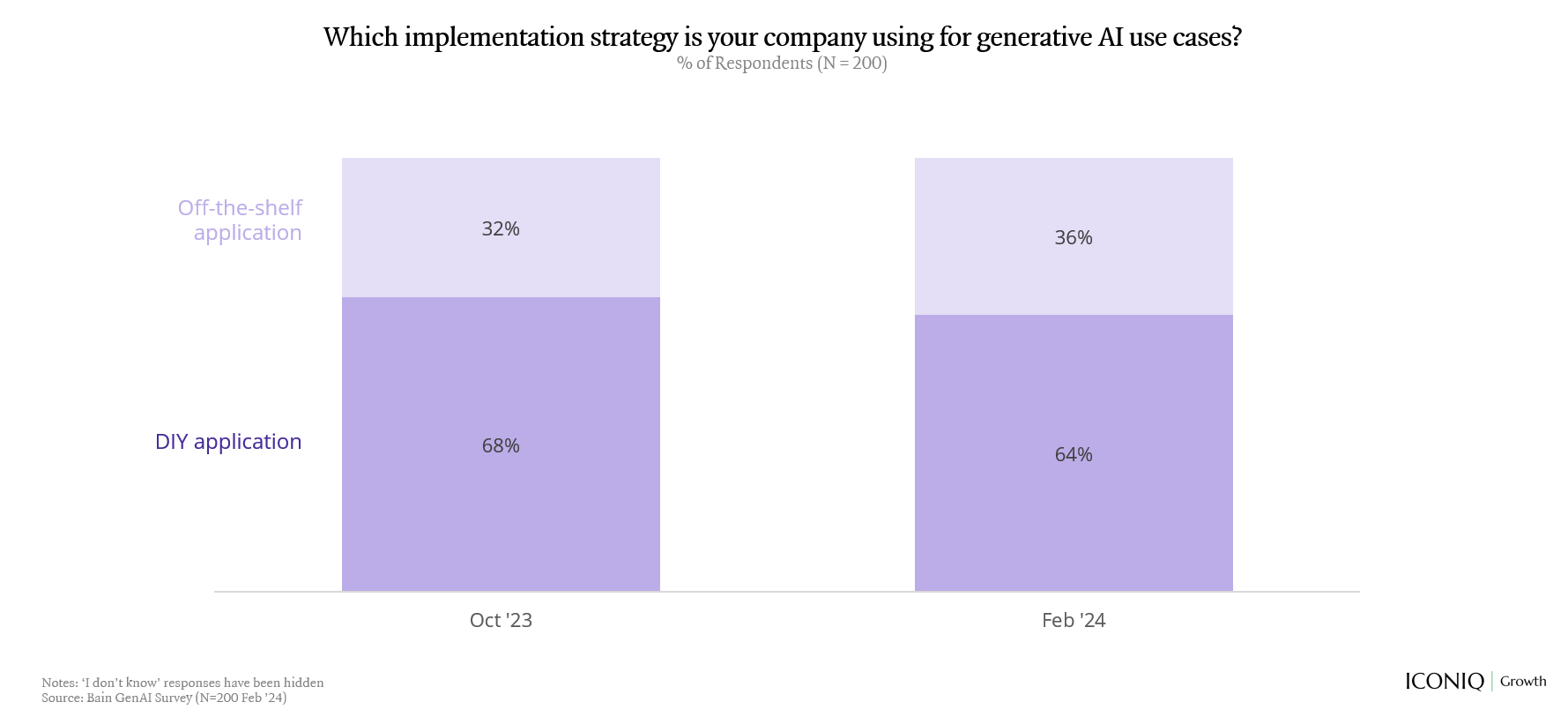
Unpacking these trends further suggests this build vs. buy dynamic varies significantly based on the use case. For example, off-the-shelf applications were more popular for improving software development due to the mass adoption of GitHub Copilot, whereas the majority of respondents are building their own AI applications to build customer-facing products or services. We plan to explore these nuanced dynamics further and remain excited about what the shifts will suggest over time as the market matures.
“Build vs. Buy has nothing to do with tech. It’s about economics. For F5000 companies, unless you are building something that can drive revenue you are wasting time building”
- CIO of Tech Company1
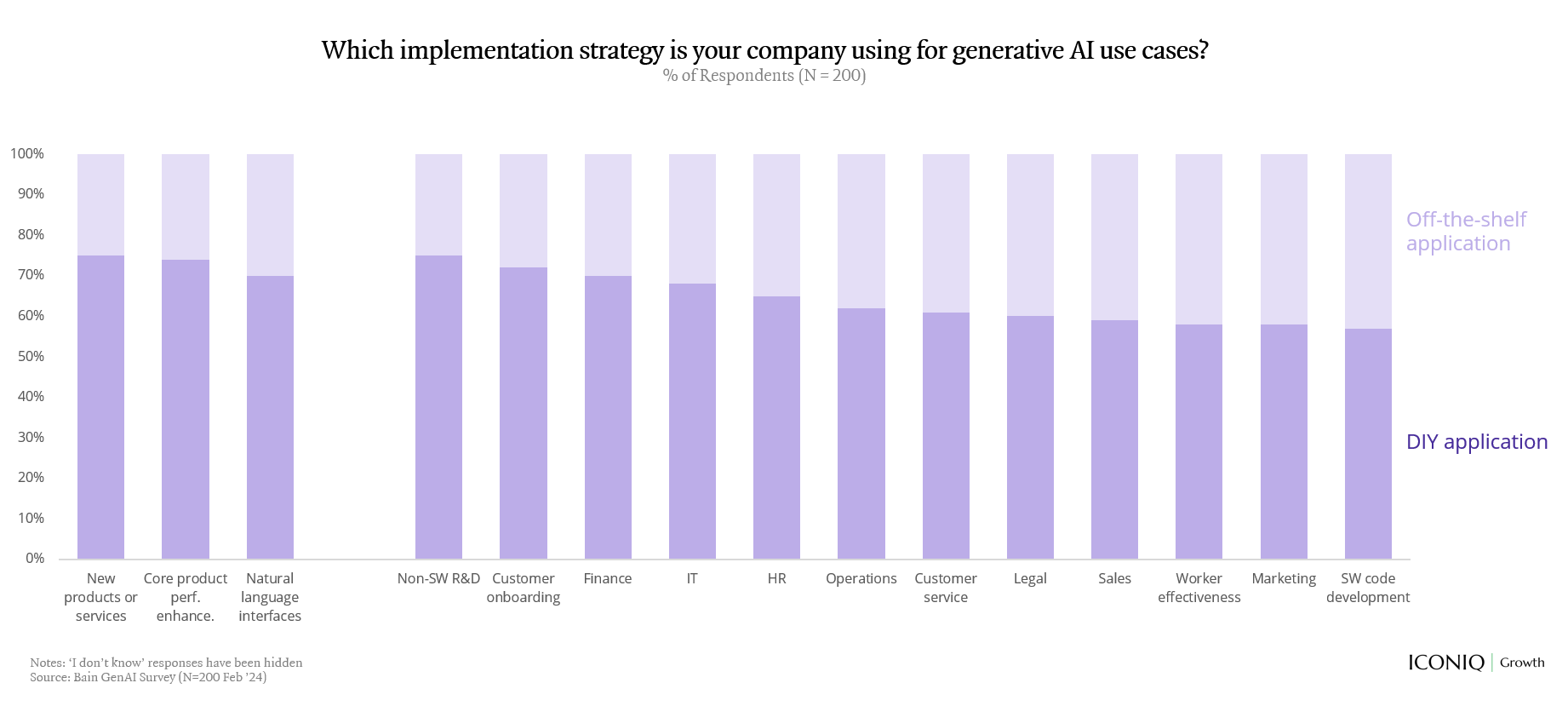
Impact and Outcomes
On average, survey respondents noted that generative AI solutions that have been live for a sufficient period of time have met or exceeded expectations in over 75% of instances. That said, there is meaningful variation in the success of these initiatives by use case, with respondents indicating a more binary distribution of outcomes in legal and non-software R&D use cases. Generative AI technologies serving customer services, sales or sales operations, and IT use cases seem to be garnering the most satisfaction to date.
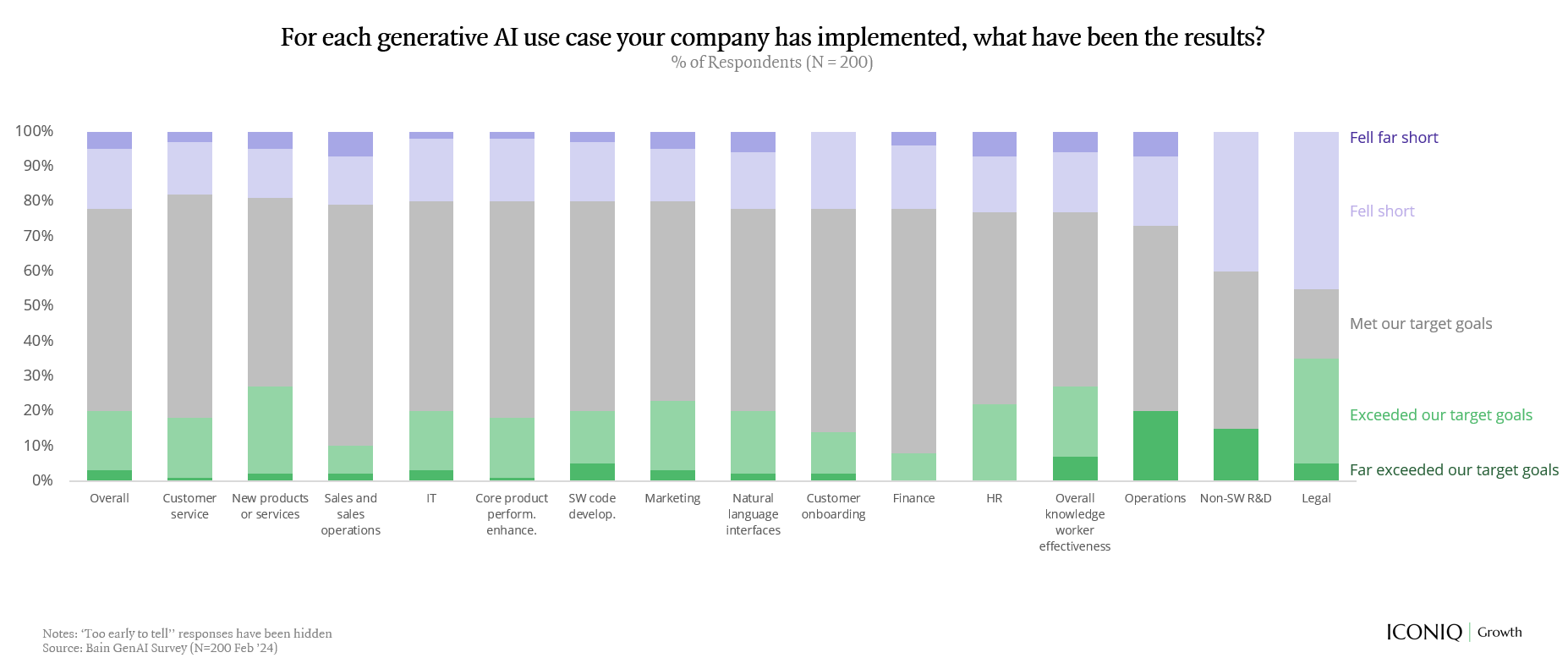
There are still meaningful challenges enterprise buyers are navigating in realizing the desired impact of generative AI initiatives. Performance, output quality, and a lack of employee understanding of how to utilize tools are the three most common shortcomings cited. Notably, a lack of understanding regarding how to use tools and low vendor/tool quality grew QoQ as a percent of total responses. For non-tech companies in particular, 57% of respondents indicated it was too early to tell when asked about the results to-date from implementing generative AI for customer-facing product use cases.
Writer’s5 recent 2024 Generative AI survey affirms these challenges, noting that in-house generative AI solutions often fall short of expectations. Results showed 61% of companies surveyed experiencing accuracy issues and only 17% rated their in-house solutions as excellent in overall performance.

What about ROI? In a recent dinner we hosted with Chief Data Officers, the consensus around the room was that measuring and quantifying the ROI of generative AI is critical. Beyond cost savings alone, decision makers are also focused on the value and outcomes that can be harder to measure, such as impact to culture, as well as knowledge and domain expansion. However, there is no clear consensus yet on best practices and the quantitative ROI to date for generative AI solutions. We look forward to diving into this topic in further detail in our upcoming research.
“We’ve already earmarked spend in our 2025 budget for a tool that is driving a ~30% reduction in time savings”
- VP Services & Partner Strategy of Tech Company1
Importantly, the total cost of ownership also impacts ROI. The Writer team explores the build vs. buy dynamic here and notes that DIY generative AI efforts have hidden costs such as development and production servers, databases, storage, and the need for an LLM and retrieval augmented generation (RAG) approach. Other factors enterprise decision makers need to consider when determining ROI are time to deployment, quality of output, security, and change management.
While buyers continue to develop frameworks to assess ROI, the market is even earlier in understanding the impact of this technology on future business operating models. Only ~20% of survey respondents report having a hypothesis around the operating model implications to leveraging generative AI at scale. As the world embraces AI, companies will also need to determine how to redeploy human talent in new ways and restructure and upskill the organization.
While there is still a lot more work to be done to maximize the impact of generative AI efforts in the enterprise, most buyers are already pointing to the fact that the adoption of such technology has resulted in defending or gaining market share, as well as increasing pricing power.

What's Next?
Generative AI brings with it significant promise to augment product offerings and improve internal productivity, but also challenges for large organizations trying to implement AI. Leaders must develop clear roadmaps, overcome technical and skill barriers, and answer the pervasive questions of how to quantify ROI from AI initiatives. Moving forward, it will be crucial for organizations to not just adapt to generative AI, but to become adept at evolving with it, ensuring they maximize the technology’s benefits while mitigating its risks.
We are excited to share additional research on these topics in the coming months and invite you to follow along here. As this space is evolving rapidly, we would also love to connect with you to exchange perspectives, whether you are a CXO trying to figure out how to get started with adopting generative AI or a founder building generative AI solutions.
Notes
(1) Based on interviews conducted by ICONIQ Growth and conversations with CIOs and CDOs from January 2024 to June 2024 at ICONIQ Growth events.
(2) Survey respondents from Bain Q1 2024 GenAI survey included 200 CXOs leading US-based organizations across all industries including technology, financial services, healthcare/pharma, consumer goods, system integrators, advanced manufacturing, marketing, and retail.
(3) From Bain Q1 2024 GenAI survey. Survey respondents included 200 CXOs leading US-based organizations across industries listed above.
(4) From Bain Q4 2023 GenAI survey. Survey respondents included 198 CXOs leading US-based organizations industries listed above.
(5) This is an ICONIQ Growth portfolio company. For the full list of ICONIQ Growth companies, please visit our website at iconiqgrowth.com/companies
Published:
August 22, 2024